In an age where data floods every profession, we find ourselves both empowered and overwhelmed. This wealth of data is a double-edged sword: while it offers invaluable insights and opportunities for discovery, it also presents significant challenges. We often grapple with the capacity to store and analyze this data, coupled with a scarcity of trained experts equipped to harness its full potential. Nowhere is this paradox more evident than in biology, a discipline that has undergone a revolutionary transformation in the last four decades or so, thanks to its integration with technology.
As the field of biology has evolving, the need for experts to analyze the growing data has given rise to new professional titles such as bioinformatician, computational biologist, data scientist, among others. These are interchangeably used which can lead to confusion among the public and students eager to delve into realm of the data analyses regardless of the specific domain. In this post, I aim to describe the roles primarily associated with biological sciences along with few closely related titles.
Bioinformatician
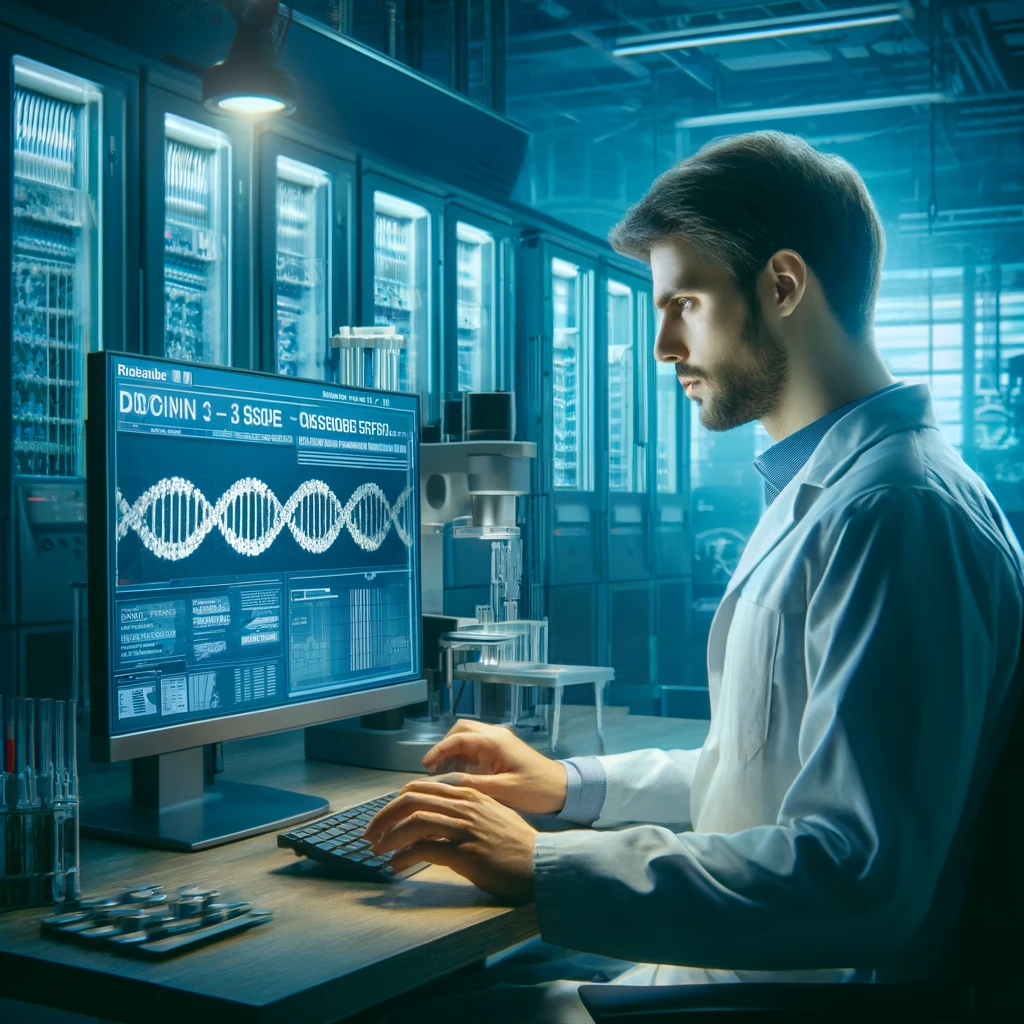
Among the titles, bioinformatician has probably been heard or used more frequently. At its core, it describes someone who navigates the intersection of biology and data, particularly biological data. Considering the vast expanse of biology as a discipline, the realm of bioinformatics is equally wide-ranging. Experts may focus on decoding genomic data, delve into transcriptomics, or explore various other ‘omics’ fields.
Following figure illustrates ‘omics’ terms based on their frequency in PubMed articles. From new millennium we see the emergence of newer areas of study expanding as years pass and more to evolve in coming years.
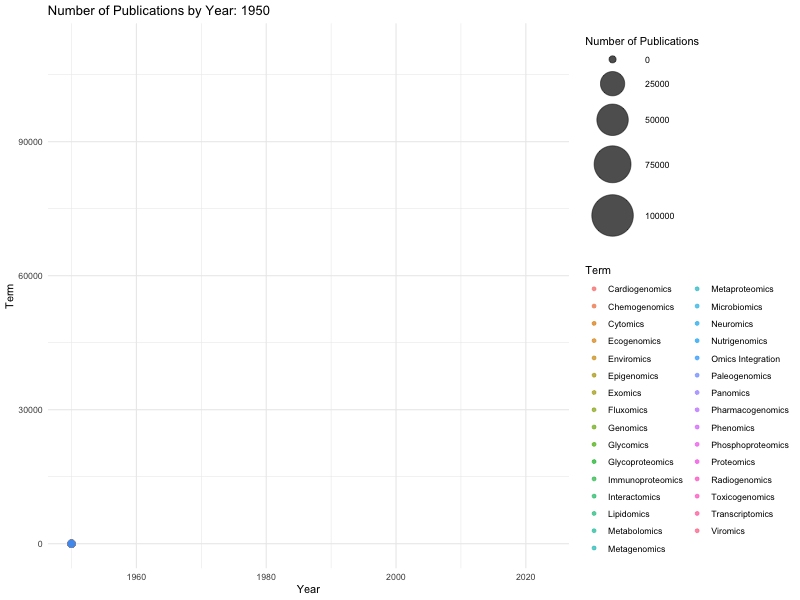
This journey into bioinformatics often starts with fundamental tasks, such as running a BLAST search—a common yet essential procedure for comparing biological sequences. However, true mastery in the field extends far beyond these initial steps. It involves a profound engagement with computational techniques, delving deeper than routine database searches. It is about harnessing computational power to address fundamental questions in biology, transforming raw data into meaningful knowledge.
Computational Biologist
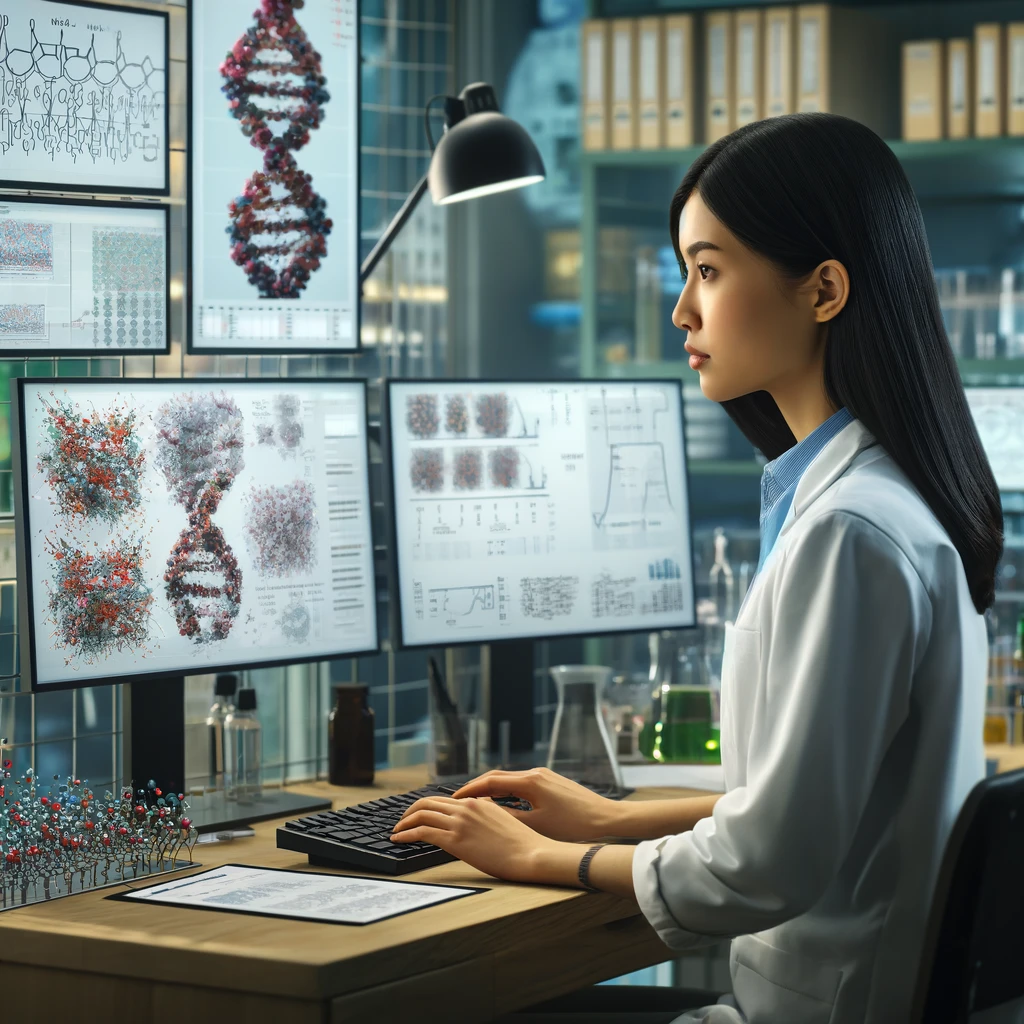
Although computational biologist and bioinformatics scientist are used interchangeably, there are differences between them. A computational biologist typically possesses a stronger computational background, applying mathematical models, algorithms, and statistical models to decipher complex biological systems and relationships. The emphasis in computational biology is more on developing computational theories, writing software, and enhancing the performance of methods implemented in such software.
Computational biologists often come from a more robust computer science background compared to bioinformaticians, who may have undergraduate degrees in biology or related fields. The field of computational biology is broad, encompassing everything from drug development to genetics. While some computational biologists, especially those with backgrounds in physics and mathematics, have made significant contributions to genomics and similar fields, their expertise in biology might not be as profound as those with formal training in biological sciences. However, they possess strong skills in tool development, mathematics, and statistics.
It is a misconception to view bioinformaticians/computational biologists merely as programmers. Although they develop computational tools and software through programming, their role transcends that of pure programmers. Depending on the organizational structure, they may collaborate with trained programmers or software developers to produce software, highlighting their interdisciplinary and multifaceted contributions to scientific research.
Data scientist
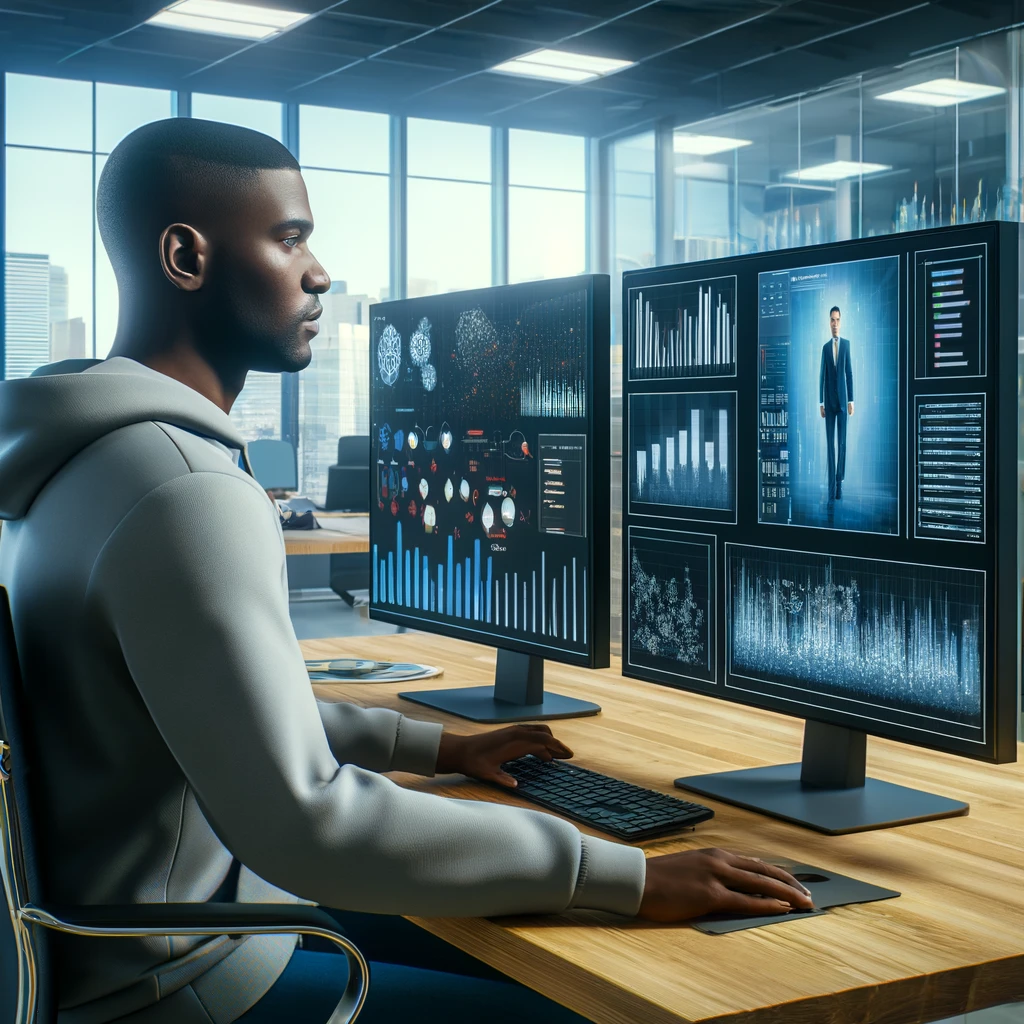
Data scientists wield a multifaceted skill set to scrutinize data from various domains, standing at the crossroads of mathematics, statistics, and computer science. They operate in a wide array of fields, and while their role may echo other positions discussed, it is their unique combination of skills that sets them apart. Depending on their educational and professional backgrounds, data scientists might not possess specialized knowledge in certain domains, such as bioinformatics, unless they have specific training in biology. Nevertheless, they employ statistical tools similar to those used by biostatisticians, highlighting the versatility of their expertise. A hallmark of data science is the development of predictive models and algorithms through machine learning, aiming to forecast trends or behaviors. This technique is pivotal across various industries, from retail, where it might predict consumer trends in clothing, to finance, where it forecasts market movements. Beyond technical prowess, data scientists excel in cleaning and organizing data, making it amenable for analysis, and possess the communication skills necessary to distill complex insights into actionable intelligence for stakeholders across the business spectrum.
Biostatistician
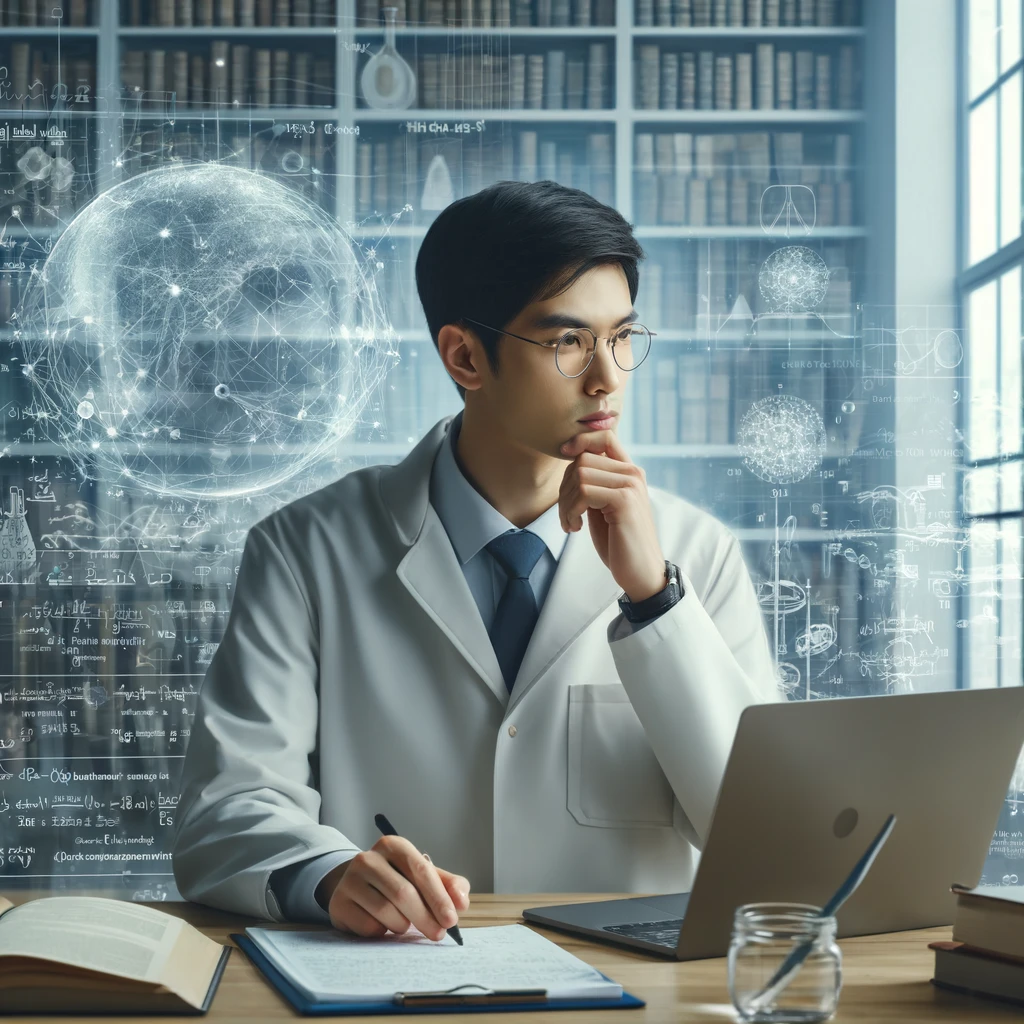
Biostatisticians are experts in applying statistical principles to biological data, a field known as biostatistics or biometry. They leverage mathematical and statistical methods to address complex questions in biology, medicine, and public health. Their crucial role extends beyond designing optimal studies; it involves calculating the power of studies to determine the required sample size for achieving desired levels of accuracy. This ensures that research findings are both scientifically valid and statistically significant. Biostatisticians are pivotal in analysing data, interpreting results, and collaborating with scientists and healthcare professionals to apply these insights to real-world health challenges. Their work is at the intersection of biology and data science, contributing significantly to evidence-based finding.
The title does not need extra explanation, it refers to people who have expertise in statistics with focus in biological data. In biostatistics (also known as biometry), people apply mathematical and statistical methods to address questions related to biology, medicine, and public health. Their role in designing optimal study is very crucial such as calculating the power of the study where they account required sample size to reach certain level of accuracy. This role is also an intersection between biology and data science.
Epidemiologist
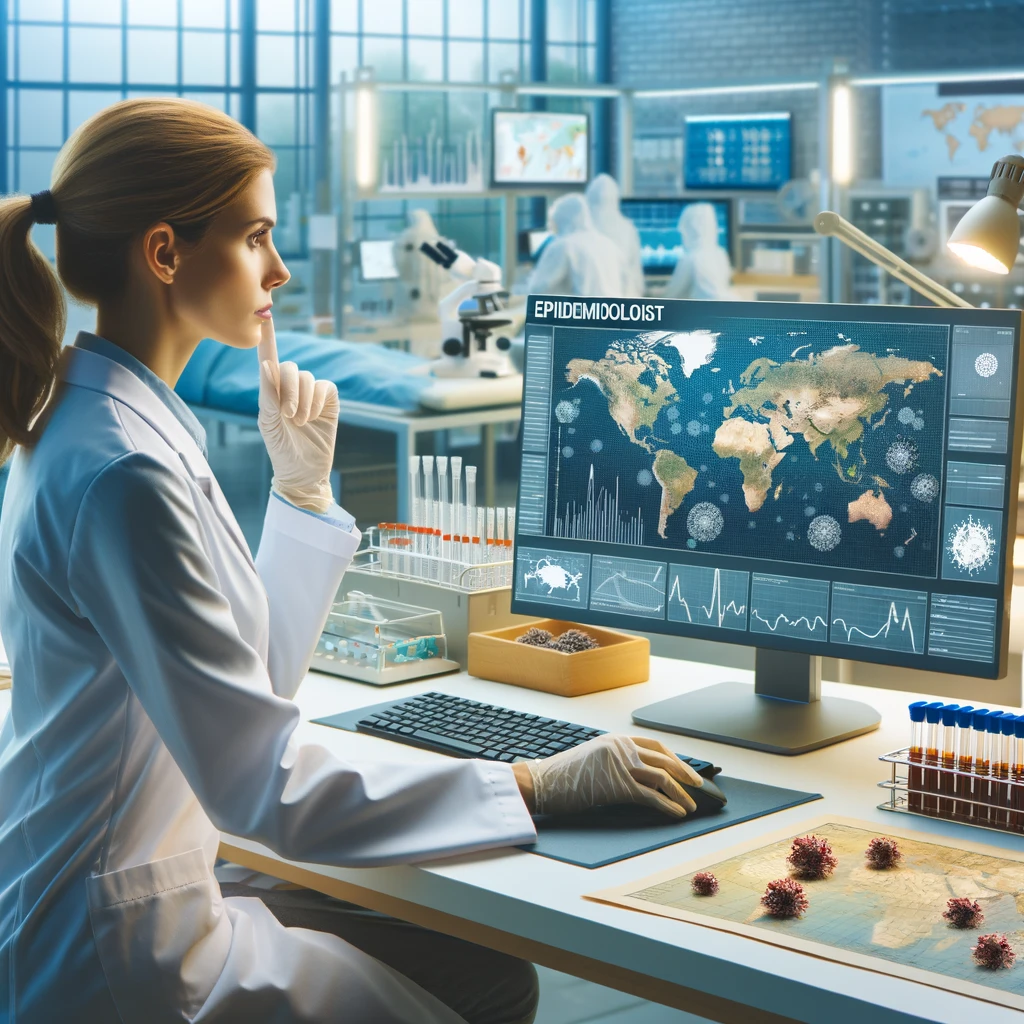
Epidemiologist have, to large extent, similar role as biostatisticians. Essentially, they use the same approaches to study diseases, health outcomes, patterns, causes, and effects in population. However, their unique contribution lies in their focus on understanding the distribution, causes, and effects of health-related states or events in specific populations. They utilize statistical tools, often developed by biostatisticians, to control and prevent disease outbreaks and inform public health decisions. Epidemiologists are indispensable in their collaboration with policymakers, applying their findings directly to design and implement effective health interventions. A prime example of their vital role is seen during the COVID-19 pandemic, where they were instrumental in controlling the outbreak through epidemiological studies and guidance.
Data Steward
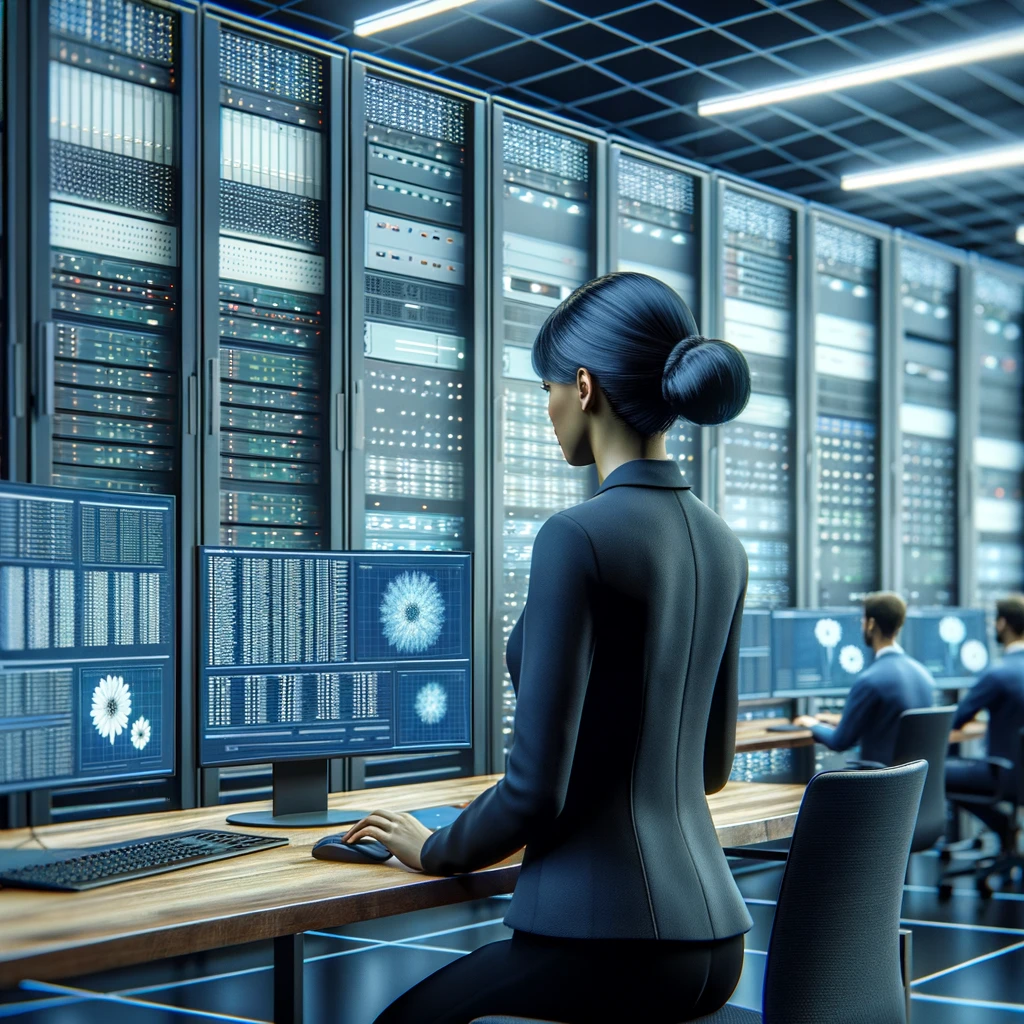
Data stewardship is an emerging profession encompassing various support functions, roles, and activities, emphasizing the professional and meticulous handling of data across all stages of a research process.
The primary responsibilities and tasks encompass a wide range, including policy advising, consultancy, operational and technical support, as well as IT-related tasks. These responsibilities can differ among research-performing organisations, and individuals in data steward roles may hold different job titles.
Data Steward can help bioinformatician, computational biologist, researchers, and other profession in given field to organise the data and help the FAIR principle and eventually publishing the results with clear data organisation.
Here I tried to highlight the key aspects of each title. However, there is no scientific discovery without a teamwork. Data analysts are very dependent on other researchers who drive the scientific questions and technicians and others who perform the experiments and generate/collect the data. I did not talk about how data analysis techniques are used in other domains of industry but to large extent is alike. The aim, in the industry, is to form a product for customers which requires good knowledge about customers interest as well as high quality products which this cannot be achieved without teamwork. At the end of the day, joined forces and collaboration of diverse minds has led and will lead to groundbreaking discoveries and valuable products.
Thanks to Angela Fuentes Pardo for inputs.
Subscribe to our newsletter!